Data lakes are next-generation data management solutions that can help business users meet big data challenges and drive new levels of real-time analytics. Their highly-scalable environment supports extremely large amounts of data.
Stored data in a data lake can be anything, from semi-structured data such as hierarchical web content, to completely unstructured data like text documents or images. This flexibility means that enterprises can upload anything from raw data to fully aggregated analytical results.
The important point to consider is that a data lake provides a single platform to save and access valuable enterprise data.
While you might have got a brief idea about what the next-gen data management solutions are all about, in our next sections, let us discuss in detail what is a data lake, how it is different from a data warehouse, and how it will impact the future of your business.
What is a data lake?
A data lake is a central storage repository that holds a vast amount of data from different sources in a raw, granular format. It can store structured, unstructured, or semi-structured data, which means data can be kept in a more flexible format for future use.
CTO of Pentaho, James Dixon coined the term ‘data lake’ that refers to the ad hoc nature of data in a data lake instead of the clean and processed data stored in traditional data warehouse systems.
Data lakes, especially the ones in the cloud, are easily scalable, low cost and often used with applied machine learning analytics. They allow users to access and explore data in their own way without needing to move the data into another system.
Now that you have understood what is a data lake, let’s do a comparative analysis between data lakes and data warehouses.
Data lakes vs data warehouse
Both data lakes and data warehouses are repositories for big data. While a data warehouse usually stores structured data, a data lake stores structured and unstructured data. Here are a few fundamental distinctions between the two that make them suitable for different scenarios.
Complex vs simple user accessibility: A data lake technology often needs an expert with a thorough understanding of the various kinds of data because it is not organized in a simplified form before storage.
A data warehouse, on the other hand, is easily accessible to both tech as well as non-tech users due to its well-defined schema. Even a member that has just started to work on a data warehouse can learn it quickly.
Flexibility vs rigidity: A data lake platform can adapt to changes quickly. Moreover, as the need for storage increases, it is easier to scale the servers on a data lake cluster. However, with a data warehouse, it involves considerable resources to modify it when requirements change in the future.
Schema-on-read vs schema-on-write: A data lake technology has no predefined schema to store data in its native form. In a data lake, most of the data preparation happens when the data is being actually used.
In a data warehouse, on the other hand, the schema is defined and structured before storage. Also, most of the data preparation usually happens before processing.
Why does your business need a data lake?
As mentioned above, a data lake platform works on a principle called schema-on-read. This means there is no predefined schema into which data needs to be fitted before storage. When the data is read during processing, it is parsed and adapted into a schema as needed. This saves a considerable amount of time that would have otherwise been spent on defining a schema. This also enables data to be stored in any format.
In addition, data lakes are highly durable and low-cost because of their ability to scale and leverage object storage. They also allow data scientists and analytics experts to access, prepare and analyze data faster with more accuracy.
If still not convinced why a data lake is important for your business, consider the few benefits mentioned below.
Improved customer interactions: A data lake technology can combine customer data from a CRM platform with social media analytics to empower the business to understand the cause of customer churn, the most profitable customer cohort, and the promotions or rewards that will increase loyalty.
No more data silos: Usually, data in most organizations is stored in various locations in different ways with no centralized access management. It’s quite challenging to access such data and analyze it accurately.
A data lake breaks down these data silos and provides seamless access to the required data for faster innovation and meaningful insights. A centralized data lake eliminates data duplication and multiple security policies.
Strong foundation for AL/ML: By having a centralized repository in the form of data lakes, multiple data sets can be combined to train and deploy machine learning models to perform predictive analysis and usage of data patterns.
Data in the data lake is stored in an open format; therefore, it makes it easier for various ML/AI-based analytical services to process this data to generate meaningful insights.
A data lake can process all types of data with low latency, including semi-structured and unstructured data like video, audio, and documents that are critical for modern machine learning and AI-based use cases.
Quality data: Due to the processing power of data lakes and the tools used, various departments can have access to quality data. This is because data lakes leverage large quantities of data and deep learning algorithms to arrive at real-time decision analytics.
Versatility and scalability: Unlike the traditional data warehouse, data lakes offer relatively inexpensive scalability. Data lakes make use of a scalability tool Hadoop, which leverages the HDFS storage to handle a growing amount of data. It is also versatile as it can be used to store both structured and unstructured data from diverse sources.
[Also Read: A complete guide on data science and analytics for business]
What are the different types of data lakes?
Data lakes can reside in the cloud, on-premises, and across multiple cloud hyperscalers such as Google Cloud or Amazon Web Services.
By far, a cloud data lake is the most popular type of data lake that offers all the usual data lake features, but in a fully managed cloud service.
Let us dig deep into each of these types of data lakes that can be used for your data management system:
1. On-premise data lake: An on-premise data lake including all its hardware, software, and processes are managed by in-house IT engineering resource. This approach has a higher capital expenditure and requires more commitment.
2. Cloud data lake: In a cloud data lake, the on-premise infrastructure is outsourced. A cloud data lake is a cloud-hosted centralized repository that allows you to store unstructured data and structured data at any scale. This approach requires a higher operational expenditure commitment, but businesses can scale more easily along with other benefits such as cost-effectiveness.
3. Hybrid data lake: Some companies choose to maintain both on-premise and cloud data lakes concurrently. This situation is generally seen during migration scenarios from on-premise to the cloud.
4. Multi-cloud data lake: In a multi-cloud data lake, two or more cloud offerings are combined. For instance, a business may use both Azure and AWS to manage and maintain cloud data lakes. This requires greater expertise to ensure these disparate platforms communicate with one another.
Data lake architecture
No matter how much data is present in a data lake, it is going to be of little use if you lack the means to utilize it effectively. Therefore, implementing proper data lake architecture is important for organizations to obtain optimal results from their data.
Data lake architecture usually consists of the following layers:
Ingestion layer: This layer ingests raw data into the data lake. The data can be ingested in real-time or batches and is organized in a logical folder structure. The ingestion layer can accommodate data from different external sources such as IoT devices, wearable devices, and social networks.
Distillation layer: The layer converts the data stored by the ingestion layer to structured data for further analysis. Raw data is converted into structured data sets and then stored as tables or files. The data is denormalized, cleansed, and derived at this stage, and then is uniformed in terms of format, encoding, and data type.
Processing layer: This layer runs user queries and advanced analytic tools on structured data. Processes can be run as a batch, in real-time, or interactively. Business logic is applied in this layer and data is consumed by analytical applications. This layer is also known as trusted or production-ready.
Insights layer: The insights layer is the query interface or output interface of the data lake. It uses SQL or noSQL queries to request and output data in reports or dashboards.
Unified operation layer: This layer is responsible for system monitoring and managing the system using workflow management, auditing, and proficiency management.
Data lakes – Use cases
Because data lake models provide the foundation for analytics and artificial intelligence, businesses across every industry are using them to increase revenue, save money, and reduce risk.
Healthcare: Data lakes have been used for many years in the healthcare industry. Due to the need for real-time insights and large amounts of unstructured data in healthcare, the use of data lake allows access to unstructured and structured data, which turns out to be a better fit for healthcare companies.
Transportation: Data lakes are a great source of insights due to their ability to make predictions. When we talk about the transportation sector, the predictions can help organizations reduce costs and improve predictive maintenance.
Cybersecurity: Cybersecurity has been a major challenge that every organization tries to minimize or eliminate. Any smartphones, laptops, or computing devices are vulnerable and susceptible to internal and external threats. Scam emails and viruses are becoming harder to identify.
To prevent such security breaches, organizations need to put into place proactive, disaster recovery, and business continuity plans. Data lakes provide a safe haven to house a business’ precious digital assets.
[Also Read: How to Ensure Cybersecurity in the Age of IoT]
Marketing: When it comes to marketing, data lakes help collect any crucial information, from demographic to preferences of both customers and prospective customers from disparate sources, for assisting in hyper-personalized marketing campaigns.
Data lakes also allow marketers to monitor and analyze data in real-time. This helps them receive timely information to make informed strategic decisions and build segmented campaigns.
Media and entertainment: A company offering music streaming, radio, and podcast services can increase revenue by improving their recommendation system, so users consume their service more and the company is able to sell more ads.
Take your data lake skyward with Appinventiv
Data lakes are multipurpose, agile and contain unstructured data for often undetermined use cases. They support important enterprise requirements such as accelerating analytic processing, simplifying data access, curating datasets, and providing a unified data catalog across all sources.
All this is done while avoiding the cost and complexity of traditional data warehouses. Data lakes also enable organizations to leave data where it is already managed, providing fast access to all data consumers, regardless of the tools they use.
At Appinventiv, our experts deliver enterprise-level data lake solutions to help you replace data silos with an agile, scalable platform that can collect, store, and govern raw data from across your business, making it ready for analysis.
For any further questions on what is a data lake or data analytics services, contact our professionals who will guide you through the entire process and offer you the best-in-class data lake and data management solutions. Talk to us!
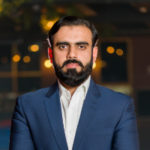
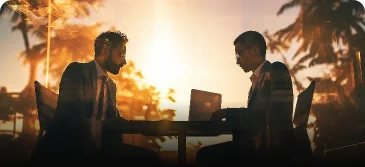
Excellence Together
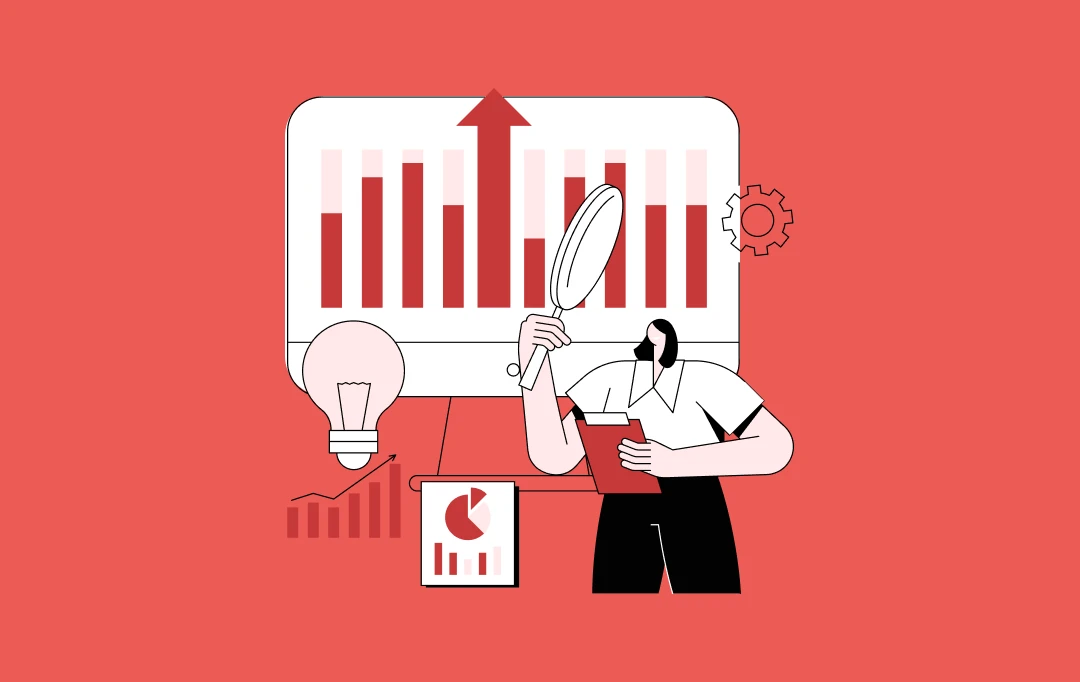
10 Ways Retail Predictive Analytics Drives Growth in Business
Earning customer loyalty and retention is more challenging than ever. With numerous choices at their fingertips, customers have elevated expectations for every interaction with a business. Additionally, the retail industry is experiencing significant financial pressures. Increased operational costs, supply chain disruptions, fierce competition, and rising marketing expenses are all contributing to the strain. Addressing these…
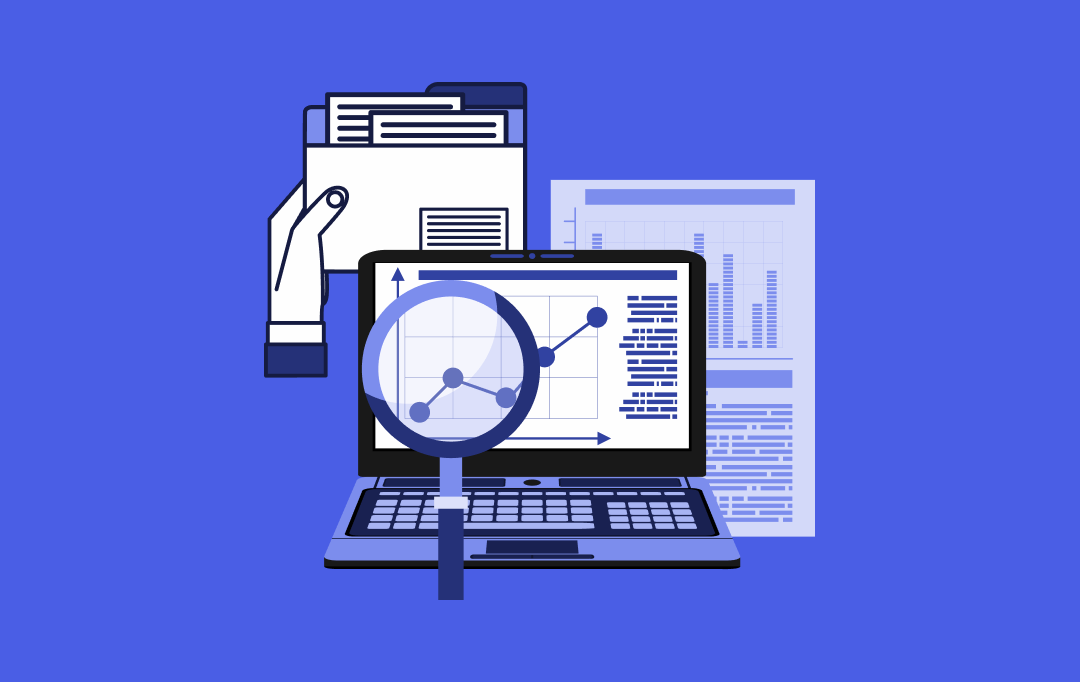
How Data Analytics is Driving Innovation in the Insurance Sector
As the insurance value chain becomes increasingly digital, insurers must swiftly understand and respond to customer needs to maintain a competitive edge. However, they often face challenges such as inaccurate risk assessments, fraud detection, and inefficient customer service. In this situation, insurance data analytics offers a critical solution, enabling insurers to leverage vast data for…
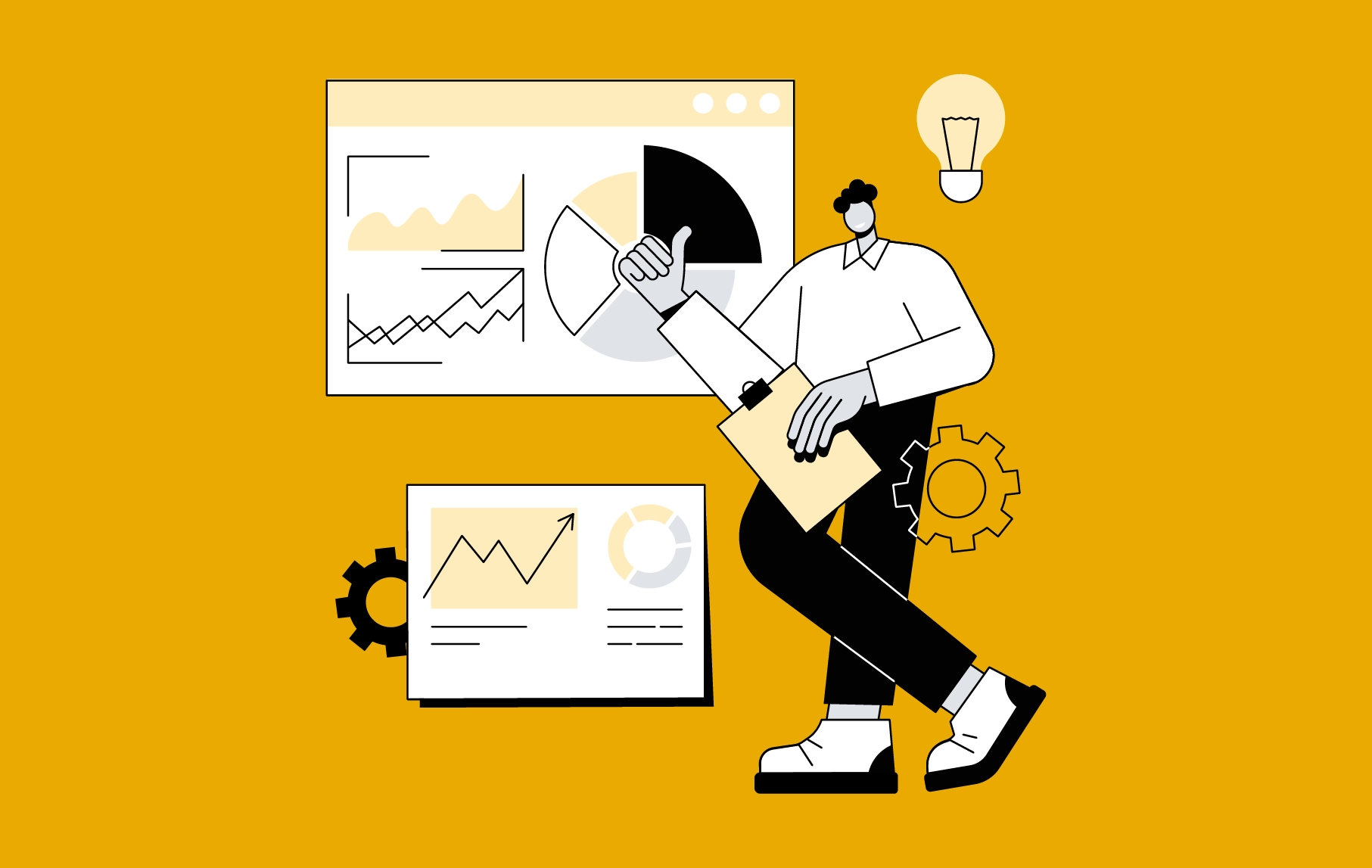