- What is Data Science and Analytics?
- Why is Data and Analytics Important for Business?
- How Does Data Science and Analytics Work?
- 1. Asking the right questions
- 2. Getting the right data
- 3. Cleaning the data
- 4. Analyzing the data
- 5. Comprehending the results
- Industrial Applications of Data Science
- Anomaly Detection
- Pattern Recognition
- Predictive Modeling
- Recommendation Engines
- Classification & Categorization
- Sentiment & Behavioral Analysis
- Conversational Systems
- Autonomous Systems
- Challenges of Implementing Data Science
- The Building Tools for Enterprise Data Science
- Data Science Technologies, Techniques, Methods
- Data Science Use Cases
- Future of Data Science
- Augmented Data Management
- Hybrid AI
- Scalable AI
- AI-as-a-Service
- Endnote
Data science is the domain that couples data-bound analytical techniques along with scientific theories to extract insights for business stakeholders. Its form, factor and magnitude enable businesses to optimize operational fluency, identify new business opportunities, and alleviate the functional performance of departments like marketing and sales. Put simply, data science imparts competitive advantages over rivals by optimizing operations with unique data throughputs.
Organizations all over the world are looking at leveraging data science and analytics for enhanced business performance. As reported by Forbes, data-driven organizations are 23 times more likely to acquire customers and 19 times more likely to be profitable.
There’s more to data than meets the eye. Therefore, with this articulative data science guide, today we would be covering a raft of topics and establish a birds-eye view of how things run in this field.
What is Data Science and Analytics?
Data science & analytics is a multidisciplinary field focused on finding deep actionable insights from large datasets. The field primarily focuses on unearthing answers to the things we do not know. Data science experts use a wide range of techniques and technologies to obtain answers to the required questions and establish solutions to problems that haven’t been thought of yet. Some such techniques and technologies include:
- Data mining
- Data engineering
- Data modeling
- Business intelligence
- Data visualization
- Predictive Analytics
- Machine Learning
- Statistical analysis
- Software programming
Why is Data and Analytics Important for Business?
Data science for business decision making is very much a reality. It is the cornerstone of business foundations in the information age. Its applications extend beyond just extrapolating insights. The curated findings help maximize efficiency. A prevalent case in point is the repurposing of data for charting buyer personas that can be (re)targeted for marketing campaigns and brand building.
Decision-makers have their hands full with figuring out the crossover benefits of data science which include but are not limited to the following:
- Fraud detection
- Financial risk management
- Cyberattack mitigation
- Industrial mechanization and management
- Advance warning systems for IT teams
Data is not just an asset, but an intercontinental currency. It can be used to optimize a company’s capabilities beginning from organizational supply chains, inventories, distribution networks, to customer service and marketing channels. The aim of this hands-on approach is to reduce capital expenditure with an outcome-oriented view of revenues.
Some of the immediate benefits of data science for businesses are increased ROIs, improved sales, streamlined operations, a quicker turnaround time for products, and increased customer engagement and satisfaction.
Quality data synthesis can lead to quantification of results and a better overview of what works and what doesn’t. Million-dollar campaigns shouldn’t be run based on whim. Instead, they should be guided by numerical evidence that outlines cost savings, business process optimization, and time-saving workflows.
While the aforementioned marquee characteristics are universal, specific value additions depend on the nature of the industry. In startups and enterprises that have a consumer-facing frontend, data can indicate the ideal target audience. Marketing divisions can utilize campaign performance data to churn hot leads and push up their conversion rates leading to better sales.
Alright, now that we have answered why data and analytics is important for business let us move on to the next section.
How Does Data Science and Analytics Work?
Data science isn’t any magic. The process involves various steps starting from asking the right questions to communicating the analysis results clearly and using AI analytics for business decision making. This workflow is described below:
1. Asking the right questions
While this might seem obvious, asking the right questions is necessary to get insightful results using data science. For instance, if you ask a question that is too ambiguous (“what type of customers are good for our business”), you will not get the desired result. Data scientists can help you better frame questions, thereby ensuring that you get the required answers.
2. Getting the right data
Once you have the right questions, a data scientist can help determine how to collect the required data for analysis. In some cases, you will already be having the data and in others, you may need to seek out new data sources.
3. Cleaning the data
Data cleaning is one of the most important aspects of the data science process. At this step, you prepare datasets for analysis by fixing or removing the data points that are corrupted, inaccurate or incomplete.
4. Analyzing the data
The core of the data science process, at this step you finally start analyzing the prepared datasets and create models. Data analysis is an iterative process and might need different parameters to be adjusted along the way.
5. Comprehending the results
Once the process of data analysis is complete, the data scientist finally explains the analysis to the stakeholders through various data visualization techniques and determines how it can be applied to achieve improved business outcomes.
Industrial Applications of Data Science
Appinventiv is one of the leading names in the field of IT solutions and advisory services offering a pool of advanced data science and analytics services including analytics consulting, data warehousing, business intelligence, and more.
In one of our projects for a leading fast food brand, KFC, we integrated advanced analytics for their team to view their app performance. Using various data visualization techniques we made it easy for the admins to track the performance and other critical areas of the app that they had to work on.
Below we’ve listed the most commonly known applications of data science encountered in organizations, which we prescribe depending upon the nature and needs of an organization.
Anomaly Detection
Anomalies are outliers in the data sets that disrupt the standards of a data cluster. This is an important application as even minutiae of deviation in data can lead to disastrous impacts.
Pattern Recognition
It is vital to a company’s existential interest to make a product offering that is relevant to the customer’s buying patterns. These patterns can be crafted after carefully sifting through big data and performing trend analysis. Pattern recognition is a fundamental method by which seasonal highs and lows can be deciphered. Speech recognition, stock trading, natural language processing, risk management, and computer vision are all applications of pattern recognition through data science.
Predictive Modeling
Flexible and nimble is always better than rigid and non-conformist. In the same vein, predictive modeling is a top-up on formulae-driven training sets. It needs to be mentioned that predictive modeling is not new, and has been around for some time. But data science ameliorates it by incorporating machine learning and deep learning. Predictive analytics in business helps close the gaps. As an example, it is called upon to be used in industrial manufacturing to minimize equipment breakdown.
Recommendation Engines
Don’t we love personalization for our favorite products?
Recommendation engines enable brands to score high on the customer satisfaction scale. They help business analysts envision the way marketing campaigns should be designed, to extend a befitting offer to the customer at the most opportune time. This helps in customer retention. Netflix and Amazon are typical examples of companies that deliver hyper-personalized experiences for customers using data science and machine learning.
Classification & Categorization
Working through structured data is rather simple. But developments in deep learning and artificial neural networks have allowed teams to manage unstructured data sets be it images, text, multimedia files, or textual documents. Through this use of data science for small business applications as well as legacy corporations, the macroeconomic ecosystem is benefitting at large.
Sentiment & Behavioral Analysis
Data analytics for small businesses and enterprises has come off age with sentiment and behavioral analysis. Through this approach, data is manoeuvered to determine the underlying sentiment of customers. As the behavioral patterns are identified the product usage trends can be tracked and monetized.
Conversational Systems
A data analytics guide cannot be complete without mentioning conversational AI-powered interfaces. These days, the building tools for enterprise data science applications include technologies that can automate mundane, repetitive tasks.
Such operations have a high dependency on human intervention. By introducing automation for such recurring KRAs, the workforce can be reallocated to more productive assignments. Intelligent agents, voice-led messenger bots, and text-to-speech interfaces are prime examples of conversational systems. Here’s an article to help you understand how bots would mold the future of work.
Autonomous Systems
Machine learning is a science that effectuates AI programs to learn and relearn from mistakes for self-correction. Such has been ML’s contribution to this industry that it has multiplied the applications of data science for business verticals. It has had a direct say in the ongoing shapeshifts in IoT and bot development.
Self-supervising robots are handling the bulk load of operations in mechanized warehouses. Self-driving cars are becoming a reality. Sooner than later, a self-sufficient world is emerging thanks to the contribution of data analytics for business owners.
(Also read: The Business Opportunities and Challenges of Implementing Analytics in Sports)
Challenges of Implementing Data Science
No introduction to data analytics for business can be considered wholesome without perusing over the challenges of this domain. To begin with, it is considered difficult to implement data analytics for business applications given the multipronged nature of specializations involved.
Often, the mind-boggling volumes of data that need to be analyzed prolong product development timelines. The talent shortage is a human resource issue in that, not every professional is adept at working with structured and unstructured data, and semi-structured data all combined. Thus, organizations need to push employees to learn from the data science course or work on smart data discovery solutions to overcome such solutions
Another challenge lies with data bias. We need to reconsider the fact that our worldview is inherently biased and this reflects in our programmatic practices. This unconscious bias seeps into data models which unfortunately may remain limited in their capacity to show results. This is probably why enterprises and organizations rely on solutions such as data lakes, enterprise data warehouses and data analytics and processing to closely mine their data.
[Also Read: Cloud data warehousing: Critical drivers and cost components]
While changing attitudes will take time, additional bottlenecks remain in finding the right kind of data. Statistics for data science and business analytics demand choosing a niche toolkit, managing the deployment of complicated analytical models, and quantifying results.
The Building Tools for Enterprise Data Science
Proceeding towards the twilight stages of our data analytics guide, it is vital that we go over the basic analytical tools and technologies that are used in app development and otherwise. The basic toolkit for data analytics for business professionals includes an equitable mix of open source and ready-to-purchase options. Some of these are mentioned below:
Spark, Hadoop, NoSQL databases
Coding languages such as Julia, SQL, Python, R, Scala, and JavaScript
Statistics for data science and business analysis requires tools such as Tableau, SAS, Apache Spark, and IBM SPSS
Since ML is an integral, unavoidable subset of data science, therefore knowledge of ML libraries such as TensorFlow, Scikit-Learn, PyTorch, and Keras proves useful
Data Science Technologies, Techniques, Methods
In this section of our data science guide, we’ll go over the crucial technologies that act as the backbone of this commercial field today. The first and foremost is Machine Learning. ML algorithms perform supervised, unsupervised, and reinforced learning methods to calibrate business dependencies. Training sets, under the strict moderation of data scientists, spew out observable data trends, insights as well as anomalies.
Then there is deep learning, which leverages ANN (Artificial Neural Networks) to sift through big data.
Data analytics for enterprise ventures constitute a heavy reliance on predictive modeling as well. For this, a sophisticated combination of machine learning, deep learning, and statistical analysis is employed to conjure relevant performance indicators. Such business processes often take the support of techniques like data classification, data regression, and data clustering.
[Also Read: Blockchain Analytics – 5 ways blockchain is impacting the data analytics industry]
Data Science Use Cases
The applications of data science for enterprises as well as small businesses are only limited by our imagination. The reach and means of this technology are branching out to help industries at scale. Let us look at some of these applications.
Entertainment: Over-the-Top streaming platforms such as Netflix are applying data science techniques to perform sentiment analysis of their subscribers. This helps in delivering hyper-personalization experiences and a higher customer retention rate as viewers are served content based on their preferences.
Financial Services: Customer portfolios go through a heavy-handed analysis through training models so as to squelch out imposters, fraudulent transactions, carry out risk management, and identify potential openings for upselling products.
Healthcare: This sector is trying out multiple approaches to tap into AI, some of which you’d find in this article. The impact of data science and analytics on business decision-making can be understood by the fact that there is a high-level consensus to implement computer vision in a bid to identify disease symptoms that may skip human observation.
AI and ML are lifting the hopes of medical professionals to detect life-threatening ailments at an early stage and therefore support the idea of algorithms scanning millions of patient records for relevant signs.
Manufacturing: The commercial use of data science for business leaders is evident in the fact that the tech is being widely trusted to optimize supply chains, predict equipment breakdowns and streamline distribution.
Check out this article to know how big data is used in manufacturing in detail.
Retail: Major retailers use data science and analytics to optimize inventory, manage supply chains, and architect precision-driven retargeting campaigns for outcome-oriented marketing.
Check out this article to know why your retail business should up investments in data analytics.
Logistics: Shipments and delivery routes are being optimized thanks to data science applications so that customers world over get their orders on time.
Travel: From passenger loads to airplane routes and customized ticketing platforms, AI, ML, and other subsets of data science are well-ingrained within the commercial side of businesses in the travel industry.
While these are some of the preliminary use cases which are visible to all, there are other industries that deserve an honorable mention such as cybersecurity, cloud computing, and business process outsourcing.
Future of Data Science
Drawing our guide on an introduction to data analytics for business to a close, we will now consider some of the trends that are expected to shape this industry in the immediate future:
Augmented Data Management
In simpler terms, it means utilizing the powers that be of machine learning and artificial intelligence for performance improvement. The aim is to activate and increase the scope of automation in hitherto redundant data management systems. AI will be asked to step in as an aid to humans rather than displacing their overlords.
Many companies have already started leveraging AI in its information systems. For instance, JobGet, in order to make the job seeking process time-friendly through its application took Appinventiv’s assistance in implementing the AI technology. With the use of this innovative technology, our team integrated the functionality of finding employers and employees on the app on the basis of location. This way, employees could connect with employers who are near their vicinity, thus eliminating the excess travel time.
[Also Read: Integrating AI technologies to help companies achieve their goals]
Hybrid AI
Business outcomes are intertwined with quality data. As of this date, data preparation, mining and management are predominantly carried out by humans. But with an increasing percolation of robotic process automation, there is a high likelihood that end-to-end data assignments will be off-loaded to AI.
Scalable AI
There are around 12,000 recognized AI startups. Not to mention the war for AI supremacy between the leading economies of the world namely China and the US. The Internet of Things is being broadened to reflect the Internet of Everything. With all this progress the aim of the collective human race would be to expand data applicabilities and improve lifestyle practices.
AI-as-a-Service
As outlined earlier, talent acquisition is where most organizations choke in terms of improving their AI systems. As a consequence of this, an increasing number of specialized vendors are emerging that offer different subsets of AI as a service. SaaS app development is being redefined. Robotics-as-a-Service (RaaS), Machine-Learning-as-a-Service (MLaaS), and NLP-as-a-Service are some examples that already have proof of concept.
Endnote
This brings us to a close for this guide on data science by Appinventiv. We are a data science and analytics services company that many businesses turn to for analytics enablement. We lend our analytical and software development capabilities to startups and enterprises. If you are looking for a B2B partnership and aspire to accelerate business expansion then we might have a word or two to say about it. Try us!
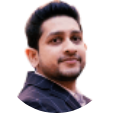
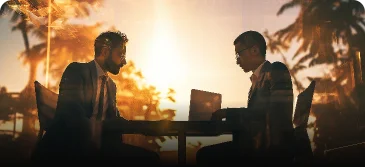
Excellence Together
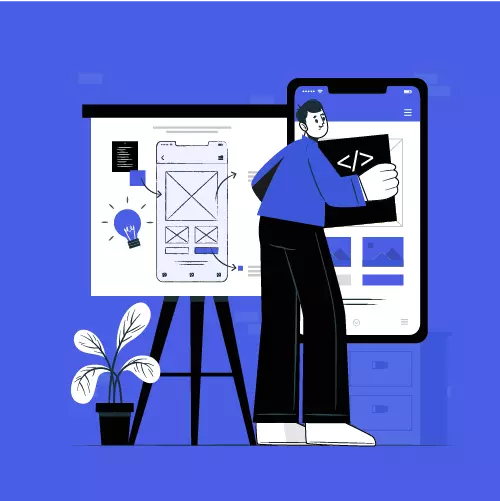
The Ultimate iOS App Development Guide for Business
The global revenue from mobile apps increased to over USD 318 billion in 2020, which was an increase of about USD 60 billion compared to 2019, as per a report by Statista. By 2025, the global mobile app market in terms of revenue is set to grow almost double to USD 613 billion, as per…
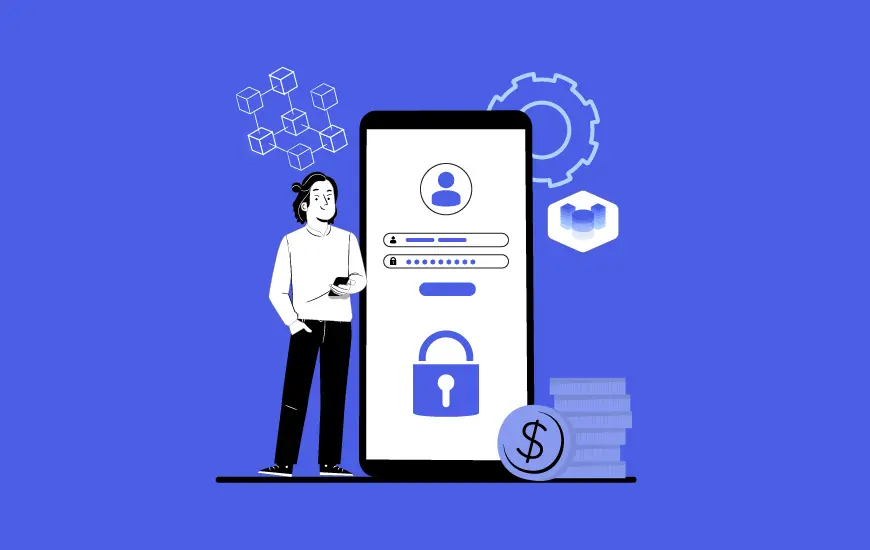
A Comprehensive Guide on Blockchain App Development Cost
The well-intentioned shift to the decentralized universe, also known as Web 3.0, has radically increased the demand for blockchain technology. The latter can revolutionize businesses and transactions, allowing business owners to create a robust, well-designed, and transparent distributed system capable of ensuring maximum ROI. Enterprises are swiftly integrating blockchain technology into their operations to revolutionize…
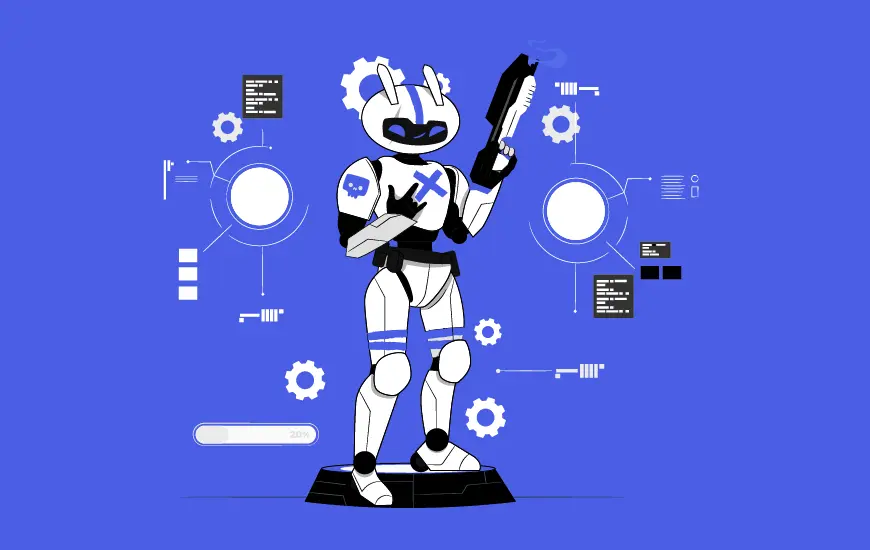