- What is Predictive Analytics in the Retail Industry All About - A Detailed Analysis
- Top 15 Ways Retail Predictive Analytics is Transforming Businesses - Use Cases and Benefits
- Demand Forecasting
- Customer Segmentation
- Personalized Recommendations
- Optimized Pricing Strategies
- Inventory Management
- Enhanced Promotional Strategies
- Improved Customer Service
- Market Trend Analysis
- Optimized Store Expansion
- Risk Management
- Enhanced Customer Retention
- Competitive Advantage
- Operational Efficiency
- Product Assortment Planning
- Supply Chain Optimization
- Real-World Examples of Businesses Using Retail Predictive Analytics
- How Does Retail Predictive Analytics Work? Key Implementation Steps
- Challenges and Solutions for Adoption of Predictive Analytics in Retail
- How Can Appinventiv be Your Retail Predictive Analytics Partner
- FAQs
Earning customer loyalty and retention is more challenging than ever. With numerous choices at their fingertips, customers have elevated expectations for every interaction with a business.
Additionally, the retail industry is experiencing significant financial pressures. Increased operational costs, supply chain disruptions, fierce competition, and rising marketing expenses are all contributing to the strain.
Addressing these challenges requires dedicated effort and strategic planning. Retail predictive analytics offers a powerful solution. By utilizing predictive analytics, retail businesses can gain critical insights to make informed decisions, optimize operations, and drive growth.
In 2023, the global retail analytics market was valued at $7.56 billion. It is forecasted to grow to $8.75 billion by the end of 2024 and surge to $31.08 billion by 2032. This represents a robust CAGR of 17.2% over the forecast period from 2024 to 2032.
Let’s dive deep into the significant role of predictive analytics in retail, demonstrating innumerable paths for businesses to witness success and growth.
What is Predictive Analytics in the Retail Industry All About – A Detailed Analysis
Retail predictive analytics involves harnessing the power of data, statistical algorithms, and advanced machine learning techniques to anticipate future outcomes in the retail industry based on historical data.
By analyzing patterns and trends from vast amounts of data, retailers can make informed decisions that optimize operations, enhance customer experiences, and drive sales.
This proactive approach allows companies to stay ahead of market changes, understand customer preferences, and respond to demands with precision, ultimately leading to a more competitive and efficient business model.
Key components of retail predictive analytics include:
Data Collection
Data collection involves gathering extensive data from sales transactions, customer interactions, and social media sources. Advanced technologies like IoT sensors and RFID tags provide real-time data on product movements. This comprehensive dataset forms the basis for accurate predictive analysis.
Data Analysis
For data analysis, experts use statistical models and machine learning algorithms to uncover patterns and trends in the collected data. Techniques like regression analysis and clustering not only predict future outcomes but also transform raw data into actionable insights, empowering businesses with the potential of data analysis.
Forecasting
Forecasting applies predictive models to anticipate customer demand, sales trends, and inventory needs. It helps in planning and resource allocation, reducing the risks of overstocking or stockouts. Accurate forecasting ensures companies can effectively meet customer needs.
Decision Support
Decision support leverages predictive insights to optimize pricing, marketing strategies, and inventory management. Predictive analytics in retail industry identify effective marketing channels and campaigns. This data-driven approach enhances overall business performance and customer satisfaction.
Top 15 Ways Retail Predictive Analytics is Transforming Businesses – Use Cases and Benefits
Predictive analytics in the retail industry has emerged as a transformative tool, offering insights that drive strategic decision-making and operational efficiency. Here are prominent predictive analytics use cases in retail elevating businesses like never before. Let’s explore!
Demand Forecasting
Predictive analytics leverages historical sales data to forecast future product demand, helping retailers optimize inventory levels, reduce stockouts, and prevent overstock situations. This demand forecasting ensures that high-demand products are always available, enhancing customer satisfaction and increasing sales.
Additionally, it provides insights into seasonal trends and market shifts, enabling retailers to adapt quickly and maintain a competitive edge. By anticipating future needs, retailers can also better manage their supply chain, reducing costs and improving efficiency.
Customer Segmentation
One of the predictive analytics retail examples is analyzing customer data to segment the customer base into distinct groups based on purchasing behavior, demographics, and preferences. This targeted approach enables more personalized marketing strategies, which improves customer engagement and retention.
Retailers can identify emerging customer trends and preferences, allowing for proactive adjustments to marketing campaigns and product offerings. By understanding the unique needs of each segment, they can create more effective loyalty programs and enhance overall customer lifetime value.
Personalized Recommendations
Advanced recommendation engines use predictive analytics to suggest products based on a customer’s past behavior, preferences, and purchasing patterns. By analyzing vast amounts of data in real-time, these systems can pinpoint exactly what a customer might be interested in at any given moment.
This level of personalization goes beyond simple product suggestions, offering a seamless and engaging shopping journey tailored to individual tastes. Personalized recommendations enhance the shopping experience, drive upselling and cross-selling, and boost customer loyalty.
Optimized Pricing Strategies
Predictive analytics helps retailers set optimal prices by analyzing factors such as market demand, competitor pricing, and historical sales data. By leveraging this technology, businesses can adapt quickly to market fluctuations, ensuring prices remain competitive while maximizing profit margins.
These data-driven insights enable the implementation of dynamic pricing strategies, adjusting prices in real-time to reflect changes in supply and demand. Dynamic pricing strategies can be implemented to maximize revenue and maintain competitiveness.
Inventory Management
Predictive analytics retail examples highlight how effective inventory management is critical for retail success. Predictive analytics provides insights into which products are likely to sell and when, allowing businesses to maintain optimal stock levels and reduce holding costs. By forecasting demand accurately, businesses can minimize overstock and stockouts, ensuring that the right products are available at the right time. This strategic approach leads to a more efficient supply chain and better customer satisfaction, as customers find what they need without delay.
Enhanced Promotional Strategies
Companies use advanced analytics in retail to identify the most effective promotional strategies by analyzing past campaign data and predicting customer responses. This data-driven approach enables marketers to craft personalized promotions that resonate with specific customer segments, boosting engagement and conversion rates. By understanding customer behavior patterns, businesses can schedule promotions for maximum impact, increasing the chances of successful campaigns and higher ROI.
Improved Customer Service
By understanding customer behavior and preferences, retailers can enhance the in-store and online shopping experience. Advanced analytics in retail enables personalized interactions and services, leading to higher customer satisfaction and loyalty.
For example, personalized recommendations based on past purchases can make shopping more enjoyable and efficient. Additionally, targeted promotions and tailored customer service can address specific needs and preferences, further increasing customer engagement.
Market Trend Analysis
Businesses can stay ahead of market trends by using predictive analytics to monitor changes in customer preferences and emerging market dynamics. This allows for proactive adjustments in product offerings and marketing strategies, keeping the business relevant and competitive.
For instance, identifying trending products early enables retailers to stock up before demand peaks. Furthermore, understanding seasonal variations and consumer behavior patterns helps in optimizing inventory and promotional strategies.
Optimized Store Expansion
Predictive analytics aids in making data-driven decisions about store expansions. By analyzing data on customer demographics, purchasing patterns, and market potential, retailers can identify optimal locations for new stores, ensuring successful expansions and maximizing returns.
For example, retailers can pinpoint high-traffic areas with underserved markets, increasing the chances of new store success. Additionally, evaluating competitors’ presence and local economic conditions can refine site selection and expansion strategies.
Risk Management
Companies can use predictive analytics to identify and mitigate risks, such as potential supply chain disruptions or shifts in consumer behavior. This proactive approach helps in maintaining business continuity and reducing potential losses.
Predictive analytics offers retail businesses a comprehensive toolkit for enhancing operational efficiency, improving customer satisfaction, and driving growth. By leveraging data-driven insights, retailers can make informed decisions that lead to sustainable success in a competitive market.
Enhanced Customer Retention
Understanding why customers churn and identifying those at risk are critical components of predictive analytics in the retail sector. By analyzing customer behavior, feedback, and transaction history, retailers can pinpoint the factors contributing to customer attrition.
With these insights, businesses can implement proactive retention strategies, such as personalized offers, loyalty programs, and targeted communication, to re-engage and retain valuable customers. By addressing issues before they lead to churn, retailers can maintain a stable customer base and enhance long-term profitability.
Competitive Advantage
One of the benefits of predictive analytics in retail is competitive advantages. Businesses that effectively utilize predictive analytics to gain a significant edge over their competitors by being able to anticipate and adapt to market changes swiftly. This capability allows businesses to stay ahead of trends, identify emerging opportunities, and mitigate potential threats.
By leveraging predictive insights, retailers can refine their strategies, enhance customer experiences, and innovate faster than their competitors. This competitive advantage is crucial in today’s fast-paced retail environment, where staying relevant and responsive is key to long-term success.
Operational Efficiency
Predictive analytics streamlines retail operations by providing actionable insights into various business aspects, from supply chain management to staffing. By forecasting demand, companies can optimize procurement and production schedules, allocating resources efficiently.
Predictive models can also assist in workforce planning by predicting peak times and adjusting staffing levels accordingly. This leads to smoother operations, reduced operational costs, and improved service levels, ultimately contributing to a better overall customer experience.
Product Assortment Planning
Predictive analytics is employed in product assortment planning to determine the optimal mix of products to stock in various locations. By analyzing customer purchase data, market trends, and seasonal variations, retailers can identify which products are likely to sell best in different stores. It reduces the risk of overstocking or understocking, which can lead to markdowns or lost sales. This approach enhances the shopping experience by ensuring customers find their desired products, thereby increasing customer loyalty.
Supply Chain Optimization
Predictive analytics in supply chain optimization involves analyzing historical data and market trends to forecast demand, manage inventory levels, and streamline logistics. By leveraging machine learning algorithms, retailers can predict potential supply chain disruptions, optimize delivery routes, and ensure timely replenishment of stock.
It enhances operational efficiency by improving delivery schedules and logistics management. Predictive models help in anticipating and mitigating risks, such as supplier delays or transportation issues, thereby ensuring a more reliable supply chain. Overall, it contributes to higher customer satisfaction by ensuring product availability and timely delivery.
Real-World Examples of Businesses Using Retail Predictive Analytics
Predictive analytics in the retail industry is a powerful tool that allows businesses to innovate and adapt to changing market conditions. Here are some real-world examples of how retail companies are using predictive analytics to enhance their operations and customer experience:
Adidas: Adidas uses predictive analytics to optimize demand forecasting, personalized marketing, and product development. By analyzing historical sales data and market trends, Adidas can accurately predict product demand, ensuring popular items are always in stock while minimizing overstock situations. In personalized marketing, Adidas leverages customer data, such as browsing behavior and purchase history, to tailor marketing campaigns to individual customers, enhancing engagement and driving sales.
Walmart: Walmart utilizes predictive analytics to optimize inventory management. By analyzing past sales data and external factors such as weather patterns and local events, Walmart can predict product demand more accurately. This ensures shelves are stocked with the right products at the right time, reducing overstock and stockouts.
IKEA: IKEA employs predictive analytics to enhance supply chain management, customer insights, and sales forecasting. By predicting product demand and understanding supply chain dynamics, IKEA ensures products are available in the right quantities and locations, reducing stockouts and overstock issues.
Predictive analytics also provides IKEA with deeper insights into customer behavior and preferences, informing product offerings and service improvements. In sales forecasting, IKEA uses historical sales data and seasonal trends to plan inventory and staffing levels more accurately.
Amazon: Amazon’s recommendation engine is a well-known example of predictive analytics in action. By analyzing customers’ browsing history, past purchases, and items in their shopping cart, Amazon predicts what products a customer is likely to buy next. This personalized recommendation system increases sales and enhances the customer shopping experience.
Macy’s: Macy’s uses predictive analytics for pricing optimization. Macy’s can dynamically adjust prices to maximize sales and profit margins by analyzing historical sales data, competitor pricing, and market trends. This real-time pricing strategy helps Macy’s stay competitive and responsive to market changes.
Nike: Nike leverages predictive analytics to improve supply chain efficiency and product innovation. Nike can predict product demand and optimize inventory levels by analyzing sales data, customer feedback, and market trends. Additionally, insights from predictive analytics help Nike innovate new products that align with customer preferences and market trends.
These examples highlight the diverse applications of predictive analytics in the retail industry, from inventory management and personalized marketing to pricing optimization and customer service enhancement. By leveraging predictive analytics, businesses can make data-driven decisions that improve efficiency, enhance customer satisfaction, and drive business growth.
How Does Retail Predictive Analytics Work? Key Implementation Steps
Predictive analytics leverages statistical algorithms, data mining, and machine learning techniques to identify the likelihood of future outcomes based on historical data. Here’s a breakdown of the steps involved into retail predictive analytics implementation:
Data Preparation
- Data Cleansing: This step involves removing inaccuracies and correcting erroneous records to ensure data quality. Missing values are also imputed to create a complete dataset.
- Data Transformation: The raw data undergoes normalization, aggregation, and the creation of derived variables. This process enhances the dataset’s usability and prepares it for analysis.
- Data Integration: Data from diverse sources is consolidated into a cohesive dataset. This integration provides a holistic view of the data landscape.
Exploratory Data Analysis (EDA)
- Pattern Discovery: Through EDA, underlying patterns and trends within the data are identified. This helps in understanding the data distribution and relationships.
- Anomaly Detection: EDA also aids in detecting anomalies or outliers that could skew the predictive model. These anomalies are addressed to maintain model accuracy.
Feature Engineering
- Feature Selection: Critical variables that significantly impact the prediction outcome are identified and selected. This reduces the dimensionality of the data and enhances model performance.
- Feature Creation: New features are created from existing data to provide additional predictive power. This step often involves domain expertise to derive meaningful features.
Model Building
- Algorithm Selection: Based on the problem type, suitable algorithms such as regression, classification, or clustering are selected. Advanced machine learning algorithms like random forests, gradient boosting, and neural networks may be employed.
- Model Training: The selected model is trained using historical data. The model learns to identify patterns and relationships within the data to make accurate predictions.
- Model Validation: The model is tested using a validation dataset to evaluate its performance. This step ensures the model’s generalizability and prevents overfitting.
Model Evaluation
- Performance Metrics: The model’s accuracy, precision, recall, F1 score, and ROC-AUC are assessed. These metrics provide a quantitative measure of the model’s predictive power.
- Hyperparameter Tuning: The model’s parameters are fine-tuned to optimize its performance. Techniques like grid search or random search may be used for this purpose.
Model Deployment
- System Integration: The predictive model is integrated into existing business processes or information systems. This allows for seamless operationalization of predictions.
- Real-Time Prediction: The model is configured to handle real-time data inputs, providing instantaneous predictions that support decision-making processes.
Monitoring and Maintenance
- Performance Monitoring: The model’s performance is continuously monitored to ensure its accuracy over time. Metrics are tracked to detect any degradation in performance.
- Model Retraining: The model is periodically retrained with new data to adapt to changing patterns. This step ensures that the model remains relevant and accurate.
Challenges and Solutions for Adoption of Predictive Analytics in Retail
Businesses face numerous challenges when adopting predictive analytics in retail, such as data quality issues, integration complexities, and a lack of skilled personnel. Additionally, ensuring data privacy and managing customer expectations can be daunting. Let’s explore the solutions to these challenges.
Data Integration
Challenge: Retailers frequently encounter difficulties in merging diverse data sources, leading to fragmented information and operational inefficiencies.
Solution: Implement comprehensive data integration platforms that seamlessly unify disparate data sources, ensuring smooth data flow and enhanced accessibility.
Data Quality
Challenge: Inconsistent and inaccurate data undermines the effectiveness of predictive analytics, resulting in unreliable insights and poor decision-making.
Solution: Utilize advanced data cleaning and validation tools to uphold high data quality standards, ensuring precise and trustworthy predictive analytics outcomes.
High Implementation Costs
Challenge: The substantial initial investment required for predictive analytics can be a significant barrier for many retailers.
Solution: Opt for scalable predictive analytics solutions that allow for phased implementation, distributing costs over time and minimizing initial financial impact.
Lack of Skilled Personnel
Challenge: The shortage of skilled personnel to manage and interpret predictive analytics tools poses a significant challenge for retailers.
Solution: Invest in comprehensive training programs and form partnerships with analytics experts to develop in-house expertise, ensuring the effective use of predictive analytics tools.
Data Security and Privacy Concerns
Challenge: Ensuring the protection of sensitive customer information and maintaining data privacy presents a significant challenge in retail analytics.
Solution: Implement robust data security protocols and stringent compliance measures to safeguard sensitive information, fostering customer trust and adhering to regulatory requirements.
Resistance to Change
Challenge: Employees may resist adopting new technologies, hindering the successful implementation of predictive analytics solutions.
Solution: Cultivate a culture of innovation and continuous improvement within the organization by showcasing the tangible benefits of predictive analytics through pilot projects and success stories.
Complex Technology Infrastructure
Challenge: An outdated and complex technology infrastructure can impede the adoption and effectiveness of predictive analytics.
Solution: Simplify the technology stack by adopting user-friendly, integrated analytics platforms that streamline operations and reduce overall complexity.
Real-time Data Processing
Challenge: Handling and processing large volumes of data in real-time is a significant challenge for retailers seeking to leverage predictive analytics.
Solution: Deploy advanced real-time data processing and analytics solutions to facilitate timely decision-making and enhance responsive retail operations.
How Can Appinventiv be Your Retail Predictive Analytics Partner
In the competitive world of retail, anticipating customer needs and market trends before they happen is crucial. This is where predictive analytics comes into play, offering the ability to forecast future trends based on vast amounts of data.
Appinventiv excels in this area, leveraging its deep expertise in artificial intelligence and data science to develop sophisticated analytics solutions. By analyzing customer transactions, social media interactions, and market movements, Appinventiv uncovers valuable insights tailored specifically to your retail business. These insights enable more informed decision-making, from inventory management to personalized marketing strategies, ensuring you remain agile and responsive in an ever-changing market.
The team at Appinventiv comprises experienced data scientists, developers, and industry experts who work collaboratively to deliver customized data analytics services. Their holistic approach ensures that every aspect of your retail operations is considered, from data collection and analysis to implementation and support, providing you with a comprehensive and seamless experience.
Contact us today to begin a transformative journey in developing state-of-the-art predictive analytics solutions for the retail industry, and revolutionize how you connect with your customers and anticipate market trends.
FAQs
Q. What is retail predictive analytics?
A. Retail predictive analytics is the use of data analysis and machine learning techniques to forecast future trends, behaviors, and outcomes in the retail industry. By analyzing historical data, such as sales, customer behavior, and market trends, companies can make informed decisions about inventory management, pricing strategies, marketing campaigns, and more. The goal is to enhance customer experiences, optimize operations, and increase profitability.
Q. How is predictive analytics used in retail?
A. Here are some prominent use of retail analytics in retail:
Inventory Management: Predictive models forecast demand for products, helping retailers maintain optimal inventory levels and reduce stockouts or overstock situations.
Customer Segmentation: Analyzing customer data to segment them into groups based on buying behavior, preferences, and demographics, allowing for personalized marketing and improved customer retention.
Sales Forecasting: Predicting future sales trends to aid in planning and budgeting, ensuring that the supply chain aligns with expected demand.
Price Optimization: Using historical sales data and market conditions to set optimal prices that maximize profits while remaining competitive.
Marketing Campaigns: The use of predictive analytics in retail stores helps in identifying the most effective marketing strategies and channels, targeting customers with personalized offers and promotions.
Customer Lifetime Value (CLV): Estimating the long-term value of individual customers to prioritize marketing efforts and resource allocation towards the most profitable segments.
Q. What are examples of predictive analytics?
A. Here are some significant examples of predictive analytics:
Recommendation Systems: Online businesses like Amazon use predictive analytics to suggest products to customers based on their past purchases and browsing history.
Churn Prediction: Entrepreneurs use predictive models to identify customers who are likely to stop purchasing from them, allowing for targeted retention strategies.
Demand Forecasting: Grocery stores use predictive analytics to forecast the demand for perishable goods, reducing waste and ensuring fresh products are available.
Fraud Detection: Credit card companies use predictive analytics to detect fraudulent transactions by analyzing patterns and anomalies in purchase data.
Dynamic Pricing: Airlines and hotels use predictive analytics to adjust prices in real-time based on demand, competition, and other market factors.
Market Basket Analysis: Retailers analyze purchase data to understand which products are frequently bought together, aiding in cross-selling and upselling strategies.
These applications illustrate the broad impact of predictive analytics across various facets of retail, driving efficiency, and enhancing customer experiences.
Q. How does predictive analytics improve inventory management?
A. Predictive analytics helps improve inventory management by analyzing past sales data, seasonal trends, and consumer behavior to forecast future demand. This enables companies to maintain optimal stock levels, reduce the risk of overstock and stockouts, and ensure that popular items are readily available, thus improving overall efficiency and customer satisfaction.
Q. Can predictive analytics help in personalizing customer experiences?
A. Yes, predictive analytics can greatly enhance personalization efforts. By analyzing customer data, such as purchase history and browsing behavior, businesses can create targeted marketing campaigns, recommend products that match individual preferences, and tailor the shopping experience to meet the unique needs of each customer, thereby boosting engagement and loyalty.
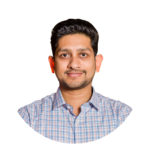
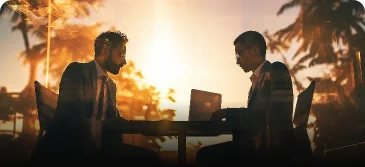
Excellence Together
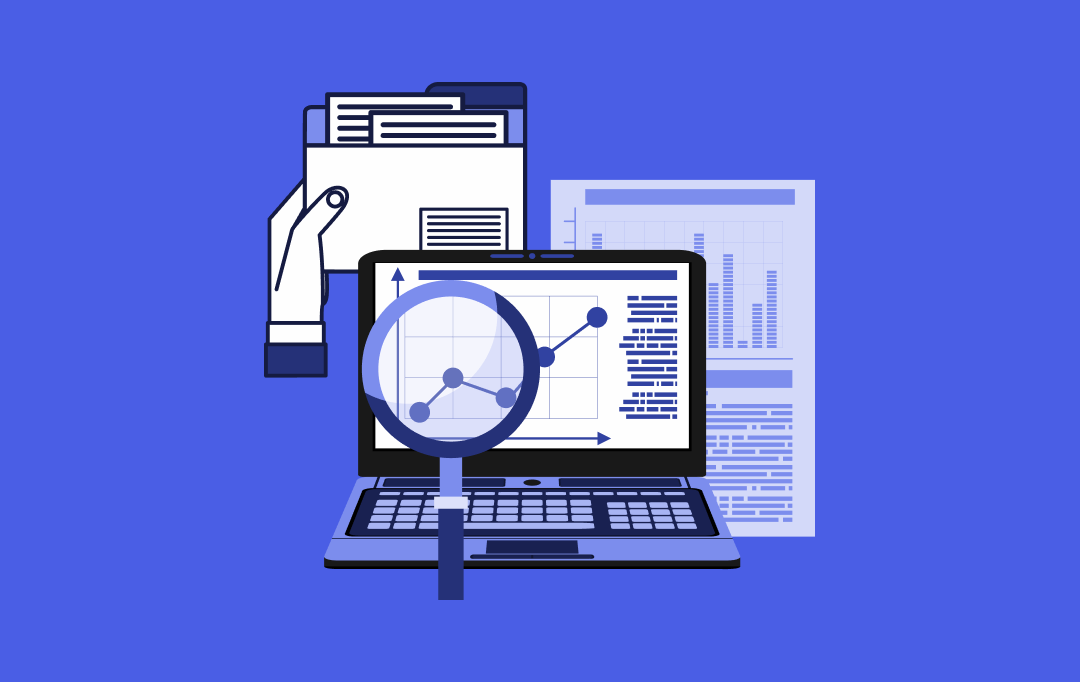
How Data Analytics is Driving Innovation in the Insurance Sector
As the insurance value chain becomes increasingly digital, insurers must swiftly understand and respond to customer needs to maintain a competitive edge. However, they often face challenges such as inaccurate risk assessments, fraud detection, and inefficient customer service. In this situation, insurance data analytics offers a critical solution, enabling insurers to leverage vast data for…
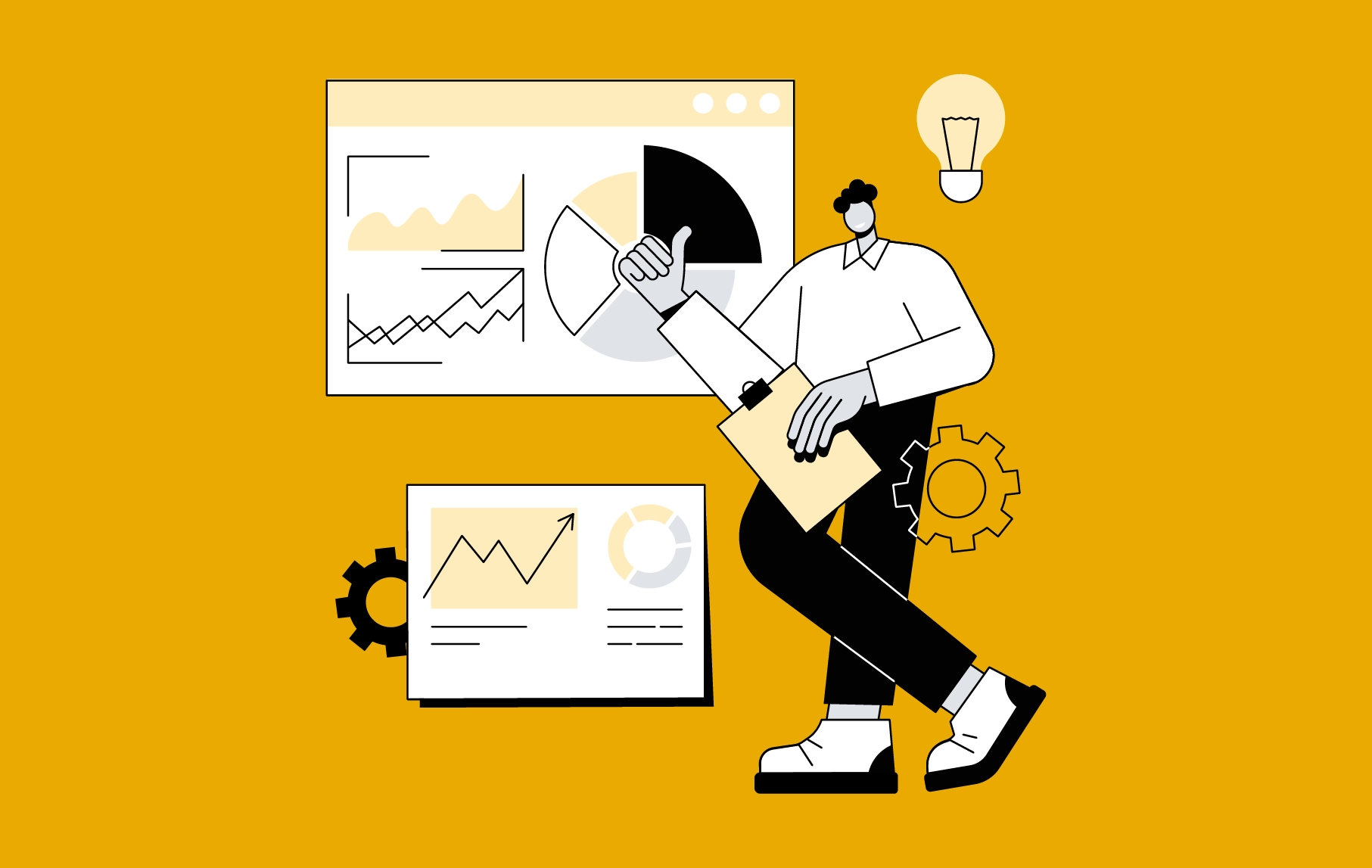
Predictive Analytics in HR - Benefits, Applications, Challenges and Real Examples
Predictive analytics in HR is revolutionizing workforce management by offering insights for smarter decision-making and strategic planning. This approach leverages data to forecast future trends, understand employee behavior, and guide informed decisions, marking a shift towards data-driven strategies in HR. The increasing reliance on this method underscores the significant growth in the HR analytics market,…
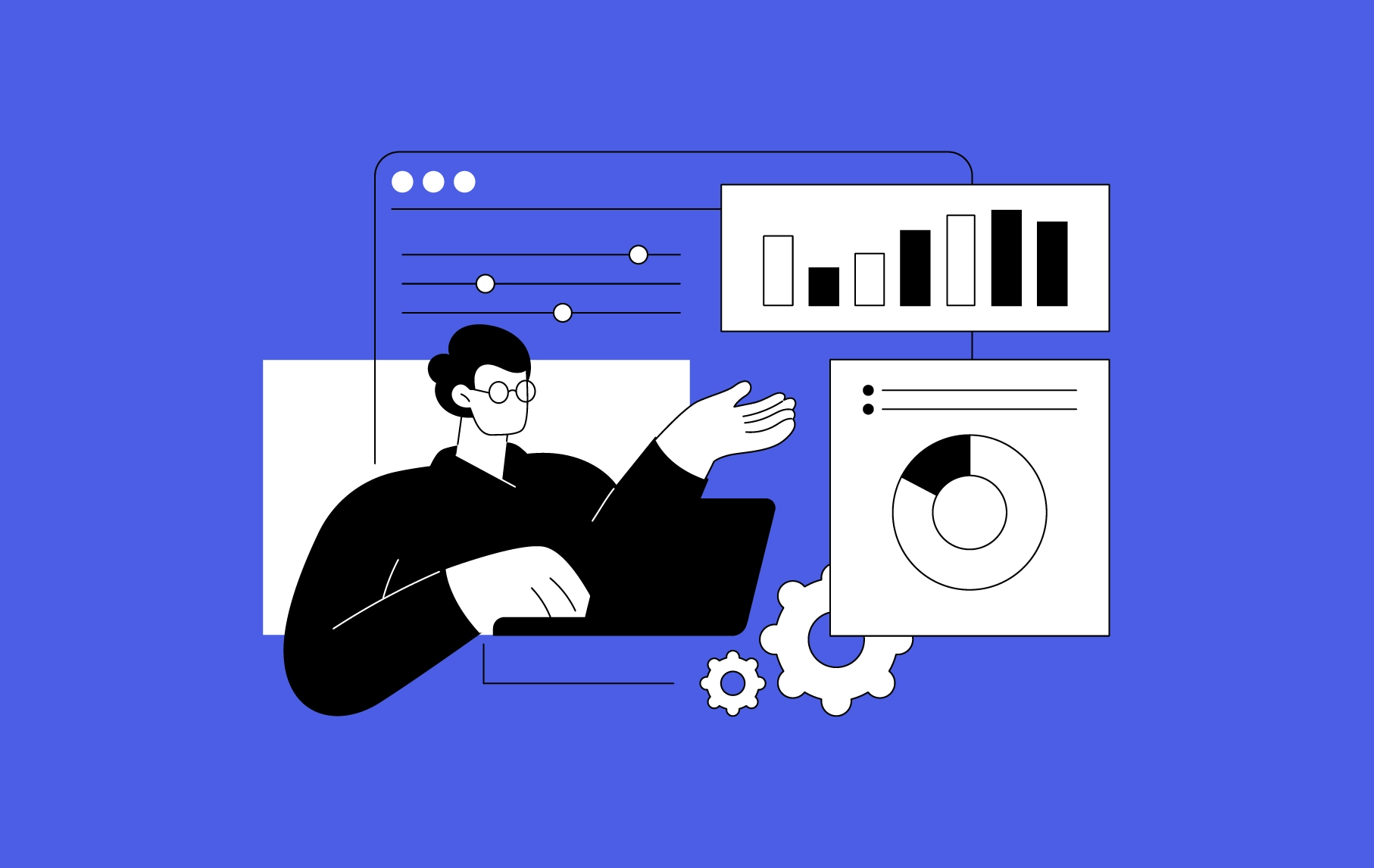